The three must haves for machine learning monitoring
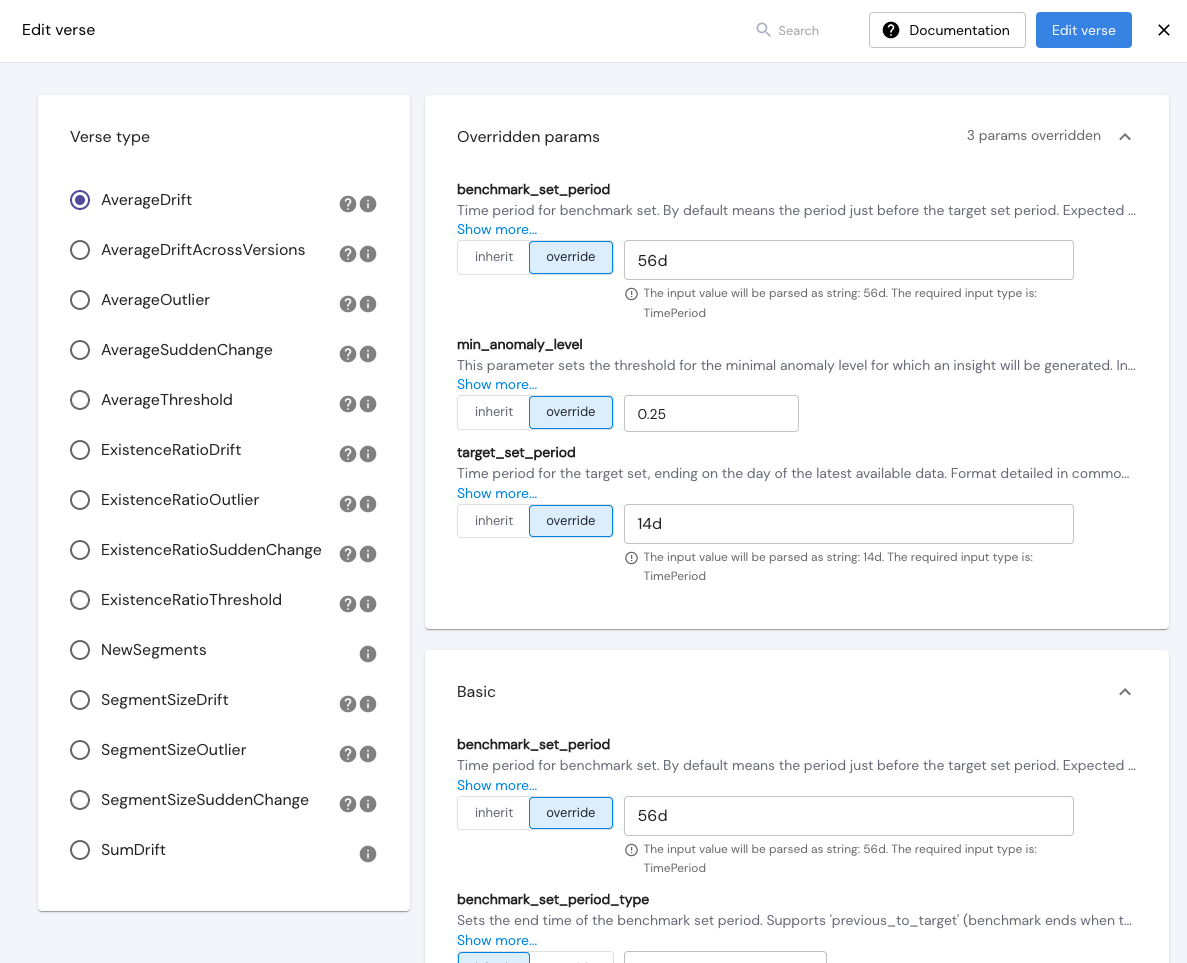
Monitoring is critical to the success of machine learning models deployed in production systems. Because ML models are not static pieces of code but, rather, dynamic predictors which depend on data, hyperparameters, evaluation metrics, and many other variables, it is vital to have insight into the training, validation, deployment, and inference processes in order to prevent model drift and predictive stasis, and a host of additional issues. However, not all monitoring solutions are created equal. In this post, I highlight three must-haves for machine learning monitoring, which hopefully serve you well whether you are deciding to build or buy a solution.