Case Study: Best Practices for Monitoring GPT-Based Applications
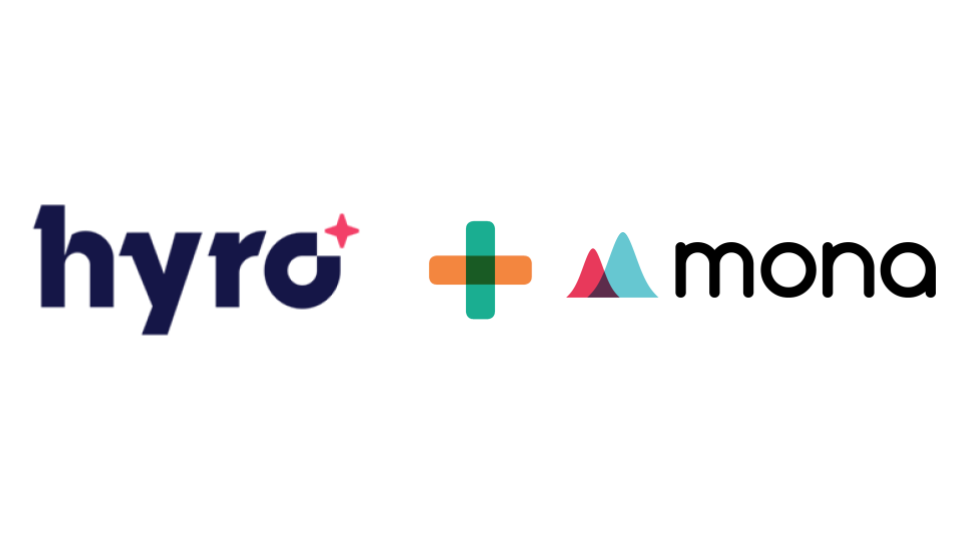
This is a guest post by Hyro - a Mona customer.
What we learned at Hyro about our production GPT usage after using Mona, a free GPT monitoring platform.
At Hyro, we’re building the world’s best conversational AI platform that enables businesses to handle extremely high call volumes, provide end to end resolution without a human involved deal with staff shortages in the call center, and mine analytical insights from conversational data, all at the push of a button. We’re bringing automation to customer support at a scale that’s never been seen before, and that brings with it a truly unique set of challenges. We recently partnered with Mona, an AI monitoring company, and used their free GPT monitoring platform to better understand our integration of OpenAI’s GPT into our own services. Because Hyro operates in highly-regulated spaces, including the healthcare industry, it is essential for us that we ensure control, explainability, and compliance in all our product deployments. We can’t risk LLM hallucinations, privacy leaks, and other GPT failure modes that could compromise the integrity of our applications. Additionally, we needed a way to monitor token usage and the latency of the OpenAI service in order to keep costs down and deliver the best possible experience to our customers.