The fundamentals of responsible AI
More than ever before, people around the world are impacted by the advancement in AI. AI is becoming ubiquitous and it can be seen in healthcare, retail, finance, government, and practically anywhere imaginable. We use it to improve our lives in many ways such as automating our driving, detecting diseases more accurately, improving our understanding of the world, and even creating art. Lately, AI is becoming even more available and “democratized” with the rise of accessible generative AI such as ChatGPT.
The dangers of unfair AI
With AI’s promise and prominence come many potential dangers. These are not just that “AI will take all of our jobs” or the sci-fi dangers where AI will “take over the world”, but more practical dangers that already impact people’s daily lives. Some dangers stem from the fact that AI systems are susceptible to changes in both real and virtual environments online. A slight difference in data can cause surprising and significant changes in an AI system’s behavior. These changes can result in fluctuating business KPIs, and also affect people’s lives in some dramatic cases, it could mean the difference between life and death (for example, self-driving cars making mistakes, or AI-driven medical diagnostics gone wrong).
Other dangers of AI are at the societal level. AI systems are less understood by the public and often even by the organizations that operate them. Algorithms may have biased behaviors, potentially leading to unfair outcomes for individuals. Given the fact that responsible and ethical AI is a field that is still in its infancy, it is quite challenging for companies to govern their AI-driven processes. These AI systems don’t have inherent biases or prejudices, they only know what they’ve been trained on in terms of data and variables. Given how much the world constantly changes, it isn’t enough to just train an AI system and unleash it into the wild without any guardrails or governance.
Some examples of real-world AI-driven processes that impact people daily and may include unanticipated biases leading to unfair processes include:
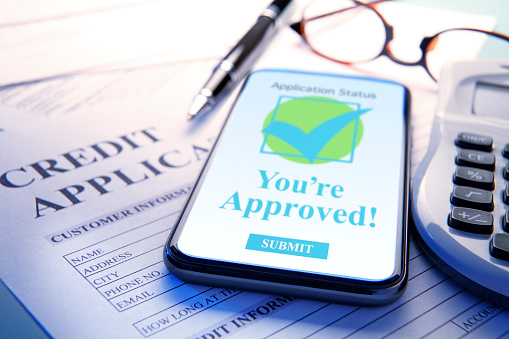
-
Getting a loan: Many lenders for both short and long-term loans rely heavily on AI to help automate the decision-making processes of creditworthiness. The AI system takes in lots of data about the applicant before ultimately making the decision to deny or approve an application and also what the terms of the loan are going to be given the data inputs. For example, an investigation by The Markup found lenders were more likely to deny home loans to people of color than to white people with similar financial characteristics. Specifically, 80% of Black applicants are more likely to be rejected, along with 40% of Latino applicants, and 70% of Native American applicants are likely to be denied.¹
-
Applying for a job: In today’s job market, it is not uncommon to see hundreds of applications submitted for an open role. Given the abundance of applications, management, and recruiting teams rely heavily on AI to help screen applicants and filter out those that may not have the desired profile for the role. Given the prevalence of these tools, The New York City Council voted 38-4 on November 10, 2021 to pass a bill that would require hiring vendors to conduct annual bias audits of artificial intelligence used in the city’s processes and tools. Companies using AI-generated resources will be responsible for disclosing to job applicants how the technology was used in the hiring process, and must allow candidates options for alternative approaches such as having a person process their application instead.²
-
Healthcare diagnosis and care recommendations: AI is now commonly used to help diagnose and give care recommendations based on patient symptoms and medical history. Overall, AI has taken monumental strides in improving healthcare but there are still instances where biases come into play. For example, a Canadian company developed an algorithm for auditory tests for neurological diseases. It registers the way a person speaks and analyzes data to determine early-stage Alzheimer’s disease. The test had >90% accuracy; however, the data consisted of samples of native English speakers only. Hence, when non-English speakers took the test, it would identify pauses and mispronunciations as indicators of the disease.³
Ongoing monitoring is imperative for responsible AI
Having better visibility into the behavior and operations of AI systems is a critical factor in making sure we avoid and mitigate the dangers associated with these systems. Comprehensively monitoring these systems allows us to ensure that quality stays up-to-par, gives us the necessary alerts when behaviors change over time, and makes AI more transparent to everyone and approachable for society.
Conclusion
In all of the above examples, proactively monitoring these processes allows organizations to observe and analyze their entire AI system while it is now serving users in the real world, enabling the detection and remediation of issues with their AI systems before they have negative impacts on the people involved. Companies have built advanced monitoring platforms that specialize in these issues and can pinpoint exactly where in the process an AI system may be unfair, giving full transparency into the factors that most influenced the system's decisions (e.g., gender, race, language, income level, and age). These monitoring platforms benefit both the company by reducing risk and ensuring compliance, and the people involved in the process by ensuring they are treated fairly.
As the importance of AI in our lives continues to evolve, the need for monitoring will only become stronger so that AI can be a force for good.
Want to see how Mona helps companies ensure fairness within AI-driven processes? Click here to read more.
Sources:
- https://www.forbes.com/sites/korihale/2021/09/02/ai-bias-caused-80-of-black-mortgage-applicants-to-be-denied/?sh=3999ec5036fe
- https://www.brookings.edu/blog/techtank/2021/12/20/why-new-york-city-is-cracking-down-on-ai-in-hiring/
- https://www.wipro.com/holmes/the-pitfalls-of-ai-bias-in-healthcare/