The issues ML model retraining won’t solve
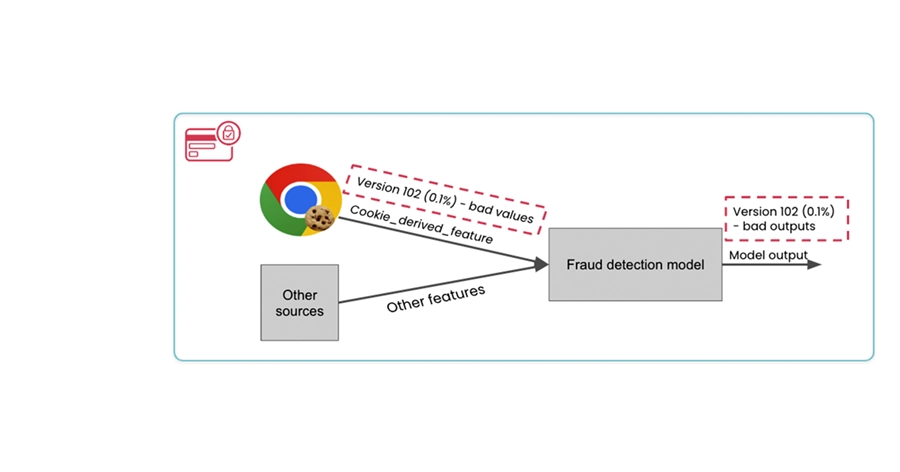
Trusting in artificial intelligence systems is not easy. Given the variety of edge cases on which machine learning models may fail, as well as the lack of visibility into the processes underlying their predictions and the difficulty of correlating their outputs to downstream business results, it’s no wonder that business leaders often look upon AI with some skepticism.