Common pitfalls to avoid when evaluating an ML monitoring solution
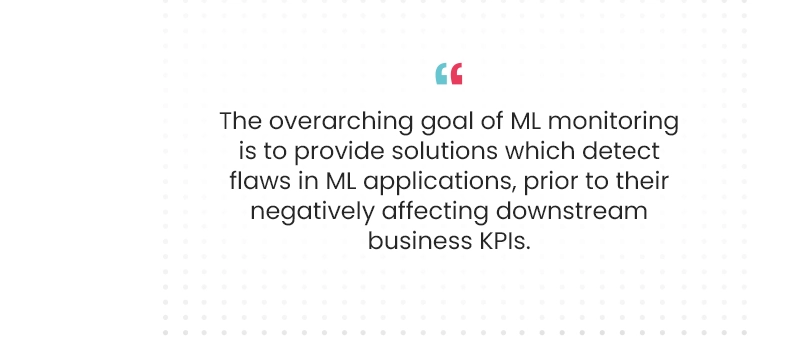
Machine learning operations (MLOps) is currently one of the hottest areas for startup investment, because while best practices for building machine learning models are relatively well understood, a great deal of innovation is being poured into devising ways to best operationalize them for production. Chief among the MLOps categories is ML monitoring. Making sense of the landscape of ML monitoring tools can be frustrating, time consuming, and just plain confusing. Our goal with this article is to chart its cartography and, in doing so, hopefully illuminate some of the common pitfalls around choosing an appropriate monitoring solution, thereby bringing order to the chaos.