So, you need AI monitoring - should you build or buy?
Before you launch a project to build an artificial intelligence monitoring system from scratch, consider whether or not this would be a good use of your resources. When does it make sense to buy instead? Let’s discuss. This post explores the advantages and disadvantages of both alternatives so that you can make an informed decision about what’s best for your organization.
Choosing an AI monitoring solution
There are many factors to consider when deciding whether to buy or build your AI monitoring system. Ultimately, it comes down to whether you can accomplish the outcomes you set out to achieve with ML monitoring, at a cost you can afford, and in a timely manner.
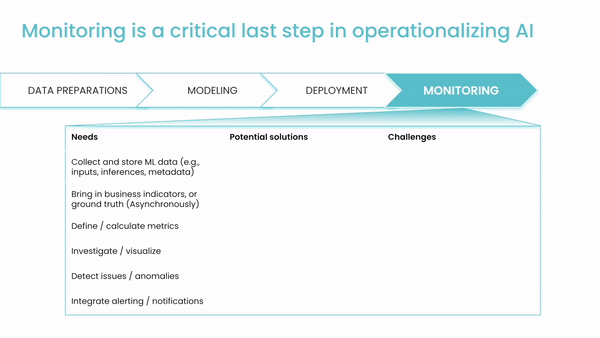
When It Makes Sense To Build:
1. Your AI program is immature; your needs are simple/basic
The need to monitor production AI often arises early, frequently before the company deployed its first ML models in the business. Data science teams universally understand the importance of visibility into data integrity and model fidelity over time, with the need for optimizations and improvements after deployment. However, in some cases, when you're still mainly experimenting or using only manual and offline processes, perhaps there is not a lot of data complexity, and perhaps the level of adoption in the business is still rather low, so the stakes are not very high. In these cases, these teams may just need a simple dashboard with basic alerting, and open source tools such as Grafana or Kibana can address these needs at this point in time.
2. Your ML monitoring needs are really niche; unlikely to be met by industry solutions
Your business might have specific requirements, such that adopting external solutions will be quite challenging. In some instances, companies may have strict security measures which will not allow other third party solutions to be installed. For example, when deploying computer vision systems in closed, secured customer physical sites; systems which effectively work independently offline and don’t allow for additional tools to be installed. In this particular example, the only viable way would be to build monitoring within the AI system.
3. You have a large central engineering team with monitoring expertise and focus
Many companies pride themselves in their strong engineering talent. However, some companies have engineering teams that are exceptionally vast, and central to the organization. These often include a wide array of domain experts including AI monitoring experts. Culturally, these companies have been building their own stack for everything (think Google, LinkedIn or even Uber). Building your own stack carries the promise of the perfect fit between your internal needs and the features built into the ML monitoring system, and possibly a dedicated team that is there to support only you. These benefits often come at a significant cost of development and maintenance, relative to the cost of external solutions.
When it Makes Sense to Buy:
1. You want to capitalize on the industry’s best practices & expertise
Building an AI monitoring platform from scratch requires tremendous expertise and a substantial staff dedicated to developing and maintaining the software, and your organization might not have enough specialized in-house talent for this. ML monitoring platforms built by monitoring providers have the insights into valuable product features and integrations, as they gather feedback from their entire slate of customers, and it’s literally their job to stay at the forefront of the model monitoring domain. Therefore, buying an AI monitoring system could be a better long-term approach to keep up with any advancements and necessary variations required.
2. You want to keep your engineers focused on the product, not the supporting infrastructure
It is also important to keep your engineers and data scientists focused on building what they do best: your product. Building an in-house AI monitoring solution would require them to shift their focus to work on supporting a tool, rather than the backbone of your company. Also, keep in mind the time commitment and resources it would take to build the solution from your current data scientists and engineers, who already have a full workload with their current day-to-day tasks.
3. You would rather be proactive and solve the ML monitoring problem sooner than later
Buying an AI monitoring system (versus building one) decreases the amount of time your company initially invests. External solutions are “ready to go”. The amount of time it takes to deploy and onboard an AI monitoring platform is significantly less than the time it would require to build an AI system from scratch. If AI is core to your business or if you have AI use cases that need to be monitored, timing may be of the essence. Making sure that your models are performing the way that they should be will save a lot of headaches for your data scientists and problems for your business.
4. You want to reduce the long term cost of development and maintenance
There’s a long-term financial benefit of buying an AI monitoring system to reduce on-going development maintenance costs. Your business may have the upfront requirements for an ML monitoring solution but as the business (and the AI program) continues to grow, the requirements may change. External monitoring solutions that are enterprise grade, should be built to scale with your AI program, and offer a fundamentally more cost effective way to support your future needs.
Choosing the right AI monitoring system for your business!
Every business owner wants to make sure that their company’s artificial intelligence monitoring system is doing its job. There are plenty of companies out there offering ML monitoring solutions, but not all of them are created equal. Before you invest in any kind of AI monitoring solution, evaluate these three factors: Proactive intelligence, flexibility, and scalability. Proactive intelligence means model monitoring is all about getting ahead of issues, so you would want your monitoring solution to continuously and systematically surface relevant problems and their root causes. Flexibility refers to being able to cater to your specific business needs and to extend to support your future needs, perhaps across multiple AI stacks and multiple business use cases. Finally, scalability is about being able to support the growth of your AI program, the transition across environments and technologies and being able to observe the AI workflows with zero interference or software performance degradation.
Overall, businesses should build an in-house AI monitoring system when it proves to offer a competitive advantage. But in most cases, buying a proven, enterprise grade AI monitoring solution built by experts tends to make more sense. If you are deciding whether to build or buy an AI monitoring solution for your business, schedule a demo to see all of the features that a full ML monitoring platform can provide for your business. We offer a live demo of our product, as well as a free consultation on AI / ML production environments.